What are we interested in?
INFORM Lab's research looks at the intersection of Infrastructure Sensing and Data Acquisition, Data Analytics and Pattern Recognition, and System Integration to enable Built Environment Performance Evaluation and Adaptive Operations for Human-Centered Sustainable and Resilient Communities.
-
Intelligent Environments
-
Adaptive Building Systems
-
Human | Context-Aware Environments
-
Artificial Intelligence for IE
-

Adaptation in Built Environment

Human-Building Interaction
-
Understanding, modeling, and facilitating interactions of users and built environment
-
Human Behavior Studies
-
Machine learning for HBI

-
Neighborhood and Urban Scale Operations and Performance Assessment
-
Interconnected Infrastructure Operations
-
Urban Participatory Sensing Network
Human-Centered Smarter Cities | Infrastructure Operational Analytics

Building Information Modeling | BE Digital Twins
-
BIM for Facilities Management
-
Built Environment Virtual Twins
-
Virtual and Augmented Reality

Data-Driven System Integration
-
Sensing and Data Acquisition
-
Emerging Technologies for Project Life Cycle
-
Machine Learning and Signal Processing for Cyber-Physical Systems
Research Efforts
** Explore the project tags for the association between project and research themes.
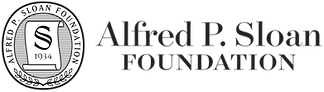

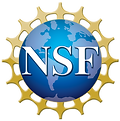
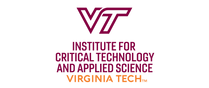

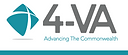
Funding Sponsors

Human-in-the-loop adaptive building operations: Location-aware applications
In human-building interaction applications, information on the location of users for service delivery is a required information element. In this direction of our research, we explore the technologies and associated data-analytics techniques to enable innovative techniques for indoor occupancy characterization and indoor positioning. Doppler radar, infrared imaging, depth cameras, and visible light communication technologies comprise the solutions that we have been developing around. Our research in this direction focuses on developing data analytics methods that use these technologies while accounting for solutions that are cost-effective, accurate, and privacy-preserving.
Read more ...
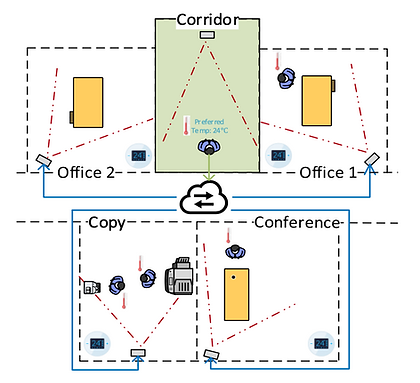
Adaption in Built Environment
Data-Driven SI
HBI
Human-in-the-loop adaptive building operations: user-dynamic-aware applications
Building systems leverage generic assumptions of user dynamics and preferences for service delivery and operation management. These assumptions have been shaped and formalized in standards over the course of the past few decades and are commonly conservative in the absence of contextual information. The advent and fast-paced development of information and communication technologies and IoT have provided the ground for a paradigm shift in human-in-the-loop operations that account for the dynamic nature of preferences and interactions in buildings. In this direction of research, we have been exploring methods at the intersection of signal processing, machine learning, and artificial intelligence, and human sciences to enable user dynamic quantification and integration for optimal and flexible control of building systems to move towards symbiotic interaction between building and their users.
Read more ...

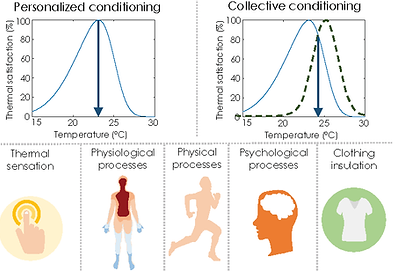
Data-Driven SI
Adaption in Built Environment
Human-Centered Smart Cities
HBI
Data-driven analysis of energy data for efficient demand-side operation and energy use flexibility
Future power distribution systems are envisioned to operate differently with the increased adoption of distributed energy resources. Accordingly, it is vital to propose novel solutions to adapt to such rapid changes to avoid performance deterioration. In this direction of our research, we develop data-driven solutions for improved operational practices of power systems based on building energy data generated by human-building interactions. The solutions aim at 1) developing autonomous systems for appliance operation identification for human-building interaction and 2) utilizing neighborhood-scale data for proposing improved automated demand-side management decisions for utilities.
Read more ...
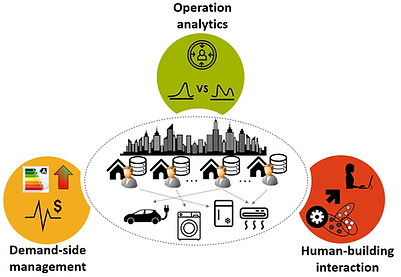
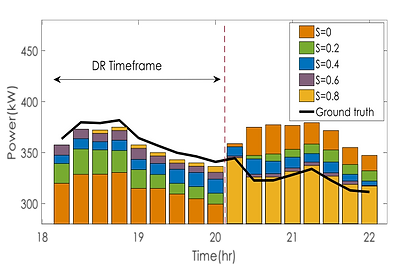
Infrastructure OA
Adaption in Built Environment
Human-Centered Smart Cities
HBI
Resilience-based urban congestion dynamics assessment through spatiotemporal data analytics
In this project, we propose using the resilience concept, which usually has been used for extreme events and disturbance to the transportation system, for high-probability low impact (HPLI) events to quantify and assess the traffic congestion, study the behavior of the roadways, and build a predictive model for identification of non-recurrent events. We look at the complex concept of resilience form a multi-dimensional perspective to consider the behavior of the roadways against disruption. Breaking down the resilience scores for different stages of the event (propagation, absorption, and recovery), enable the exploration of the potential reason in the formation of bottlenecks. To enhance the resilience of the system, we are investigating predictive model to the analysis by coupling resilience-oriented approach with machine learning as a supplementary approach that enriches the toolbox for performance-based planning and improving traveler's information systems.
Read more ...

Infrastructure OA
Human-Centered Smart Cities
Immersive virtual twins for BIM applications and human-building interaction
Through a series of experimental studies, we are investigating the characteristics of different pipelines for creating immersive virtual environments and their efficacy as virtual digital twins of environments in human-building interaction studies.
​
Read more ...
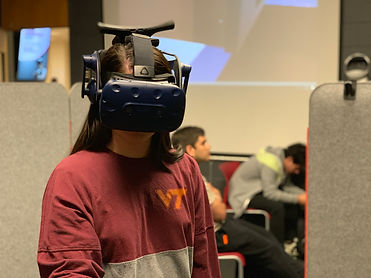
BIM | VR | AR
HBI
Energy monitoring and disaggregation
We investigate the techniques that could be leveraged to identify the interaction of users with appliances for energy monitoring and inference of the user dynamics. These methodologies have been centered around supervised and unsupervised machine learning frameworks that facilitate the deployment of these techniques in real-world applications.
​
Read more ...
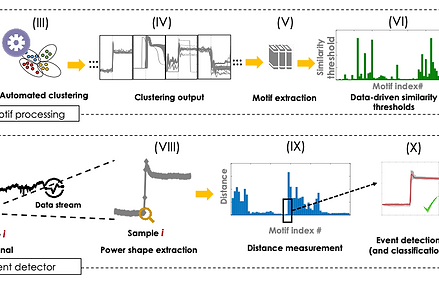

Data-Driven SI
HBI
Self-configuring machine learning frameworks for human-centered systems
The application of artificial intelligence and machine learning techniques for smart sensing and actuation in the built environment commonly calls for user intervention for in-situ configuration of algorithms. In this direction of our research, we explore techniques that help us reduce the need for user intervention in configuring the algorithms. Accordingly, we have been focusing on developing and validation of machine learning algorithmic frameworks that enable such capabilities.
Read more ...
Self-configuring machine learning frameworks for human-centered systems
The application of artificial intelligence and machine learning techniques for smart sensing and actuation in the built environment commonly calls for user intervention for in-situ configuration of algorithms. In this direction of our research, we explore techniques that help us reduce the need for user intervention in configuring the algorithms. Accordingly, we have been focusing on developing and validation of machine learning algorithmic frameworks that enable such capabilities.
Read more ...

Data-Driven SI
HBI
Infrastructure OA
Machine learning frameworks for railroad track monitoring
We have investigated innovative machine learning frameworks on large historical datasets from railroad track monitoring to facilitate defect detection in large sets of data and reduce user workload and dependence on experienced operators.
​
Read more ...

Data-Driven SI
Infrastructure OA